Artificial intelligence is rapidly changing the cybersecurity strategy and has the potential to revolutionize how organizations protect their systems and data. One of the key benefits of using AI in cyber security is the ability to analyze large volumes of data. Identify potential threats more quickly and effectively. Machine learning algorithms, for example, can be trained to identify and block malicious activity. While natural language processing can be used to analyze unstructured data such as emails and social media posts.
Another benefit of using the future of artificial intelligence in cybersecurity is the ability to automate incident response. AI-powered systems can be used to quickly identify and respond to cyber threats. Allowing organizations to minimize the damage caused by an attack. Additionally, AI can also be used to improve network security by identifying anomalies and unusual patterns that may indicate a cyber-attack.
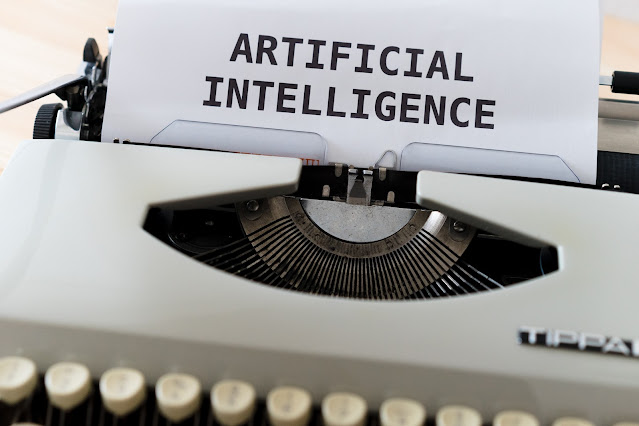
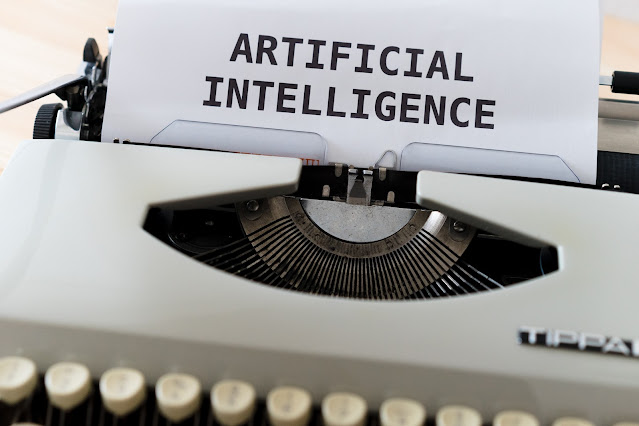
Concerns About Using AI In Cyber security
However, there are also concerns about the potential negative consequences of using Artificial in computer security. For example, AI-powered malware can evade detection by traditional security tools. AI-controlled botnets can launch distributed denial-of-service (DDoS) attacks that are difficult to defend against. Additionally, the use of AI in cyber security also raises privacy concerns. As well as the potential for the technology to be misused by malicious actors.
1. AI Powered Malware
AI-powered malware can evade detection by traditional security tools, making it difficult for organizations to protect their systems from cyber-attacks. AI-powered malware is a type of malware that uses AI engineering to evade detection by traditional security tools. These types of malware can learn and adapt to new security measures, making them difficult to detect and remove. For example, they can change their behavior and appearance to evade signature-based detection. Can use machine learning algorithms to identify and bypass security controls.
AI-powered malware can also be used to launch more sophisticated attacks. Such as targeting specific individuals or organizations and spreading rapidly through networks. To protect against AI-powered malware, organizations need to implement advanced security measures such as machine learning-based detection systems. As well as regular software updates and patches. Organizations should also monitor for unusual activity on their networks and keep an eye out for indications of a potential malware infection. Such as a sudden spike in network traffic or changes in system performance.
2. AI Controlled Botnets
AI controlled botnets can launch distributed denial-of-service (DDoS) attacks that are difficult to defend against. AI-controlled botnets are networks of infected devices, such as computers or IoT devices, controlled by a malicious actor using AI. These botnets can be used to launch a variety of cyber-attacks, including Distributed Denial of Service (DDoS) attacks. Which can overload a website or network with traffic, making it unavailable to legitimate users. Unlike traditional botnets that are controlled by a single command-and-control server. AI-controlled botnets can adapt and evolve, making them more difficult to detect and disrupt. For example, they can change their behavior and communication patterns to evade detection and can use machine learning algorithms to optimize their attack strategies. Additionally, they can also be used to launch more sophisticated attacks. Such as targeted attacks on specific individuals or organizations.
To protect against AI-controlled botnets, organizations need to implement advanced security measures. Such as machine learning-based detection systems and regular software updates and patches. Additionally, organizations should also monitor for unusual activity on their networks. Keep an eye out for indications of potential botnet infection, such as a sudden spike in network traffic or changes in system performance. Additionally, organizations should also secure IoT devices and ensure they are not connected to the internet or properly secured. This will reduce the risk of these devices being used in a botnet attack.
3. Lack Of Transparency And Explainability
AI-based systems can be complex and difficult to understand, which can make it challenging to assess their security and identify vulnerabilities. These systems can be complex and difficult to understand, which can make it challenging to assess their security and identify vulnerabilities. Additionally, it can also be difficult to understand how and why an AI-based system made a particular decision, which can make it difficult to identify and correct errors. This lack of transparency and explainability can create challenges for organizations that need to comply with regulations or demonstrate the security of their systems to customers and other stakeholders. Additionally, it can also create challenges for incident responders who need to quickly identify and respond to cyber threats.
To address these concerns, organizations can use techniques such as model interpretability, which can help to better understand how an AI-based system is making decisions. Additionally, organizations can also use techniques such as adversarial examples, which can help to identify and correct errors in AI-based systems. Furthermore, organizations can also use techniques such as explainable AI (XAI), which can provide an explanation of the reasoning behind an AI-based system’s decision, and help to ensure that the system is operating as expected.
Overall, organizations must be aware of the potential lack of transparency and explain the ability of AI-based systems and take steps to mitigate this risk. This includes implementing security measures such as model interpretability, adversarial examples, and explainable AI (XAI) to better understand and control the decision-making of AI-based systems.
4. Bias And Errors
AI-based systems can be trained with biased data, which can result in errors and false positives.
5. Privacy Concerns
The use of AI-powered surveillance systems raises concerns about privacy and civil liberties.
6. Cyber-Physical Systems Security
AI-based systems can control physical systems, such as self-driving cars, drones, and robots. Which can pose a significant risk if they are compromised by cyber-attacks.
7. Autonomous Systems
The use of autonomous systems for incident response raises questions about accountability and decision-making in the event of a cyber-attack.
8. Weaponization
AI-based systems can be weaponized by malicious actors. Which can enable them to launch more sophisticated and deadly attacks. Organizations must be aware of these concerns and take steps to mitigate the risks associated with using AI in cyber security engineering. This includes implementing security measures such as encryption, access controls, and monitoring systems, as well as staying informed about the latest developments in ai to ensure they can effectively protect their systems and data.
Conclusion
In conclusion, AI has the potential to impact significantly Computer security software in positive ways by improving threat detection, incident response, and network security. However, organizations must also be aware of the potential negative consequences of using AI in computer and network security, such as the potential for misuse by malicious actors, and the ethical implications of the technology.